MODERATOR:
Jonathan Schultz
Director, Journal Operations
American Heart Association
Cantonsville, Maryland
SPEAKERS:
Robyn Mugridge
Publishing Partnerships Manager
Frontiers
United Kingdom
Hannah Hutt
Product Manager
Frontiers
Lausanne, Switzerland
Jennifer M Chapman
Senior Managing Editor
American Society of Civil Engineers
Reston, Virginia
Daniel Evanko
Director of Journal Operations and Systems
American Association for Cancer Research
Philadelphia, Pennsylvania
REPORTER:
Ellen F Lazarus
Freelance technical and medical editor
Lazarus Editing and Consulting, LLC
Washington, DC
Journal publishers are increasingly turning to artificial intelligence (AI) technologies to address the challenges and complexities of scientific editing and publishing. The types of challenges addressed are limited only by the imagination and ingenuity of the AI system designers and end-users. In this session, Robyn Mugridge and Hannah Hutt (Publishing Partnerships Manager and Product Manager at Frontiers), Jennifer Chapman (Senior Managing Editor at the American Society of Civil Engineers [ASCE]), and Daniel Evanko (Director of Journal Operations and Systems at the American Association for Cancer Research [AACR]) presented 3 cases studies involving specific journal publication challenges and the proprietary and public AI-assisted editorial tools used to solve them.
Jonathan Schultz, Editor-In-Chief of Science Editor and Director of Journal Operations at the American Heart Association, started the session with a nontechnical introduction to the terms AI, machine learning, data/text mining, and natural language processing. (This session was generally limited to the application of the tools and did not cover technical aspects of their development and implementation.)
Case 1: Addressing Shared Challenges in Journal Publication by Developing an AI-Assisted Editorial Tool
The Artificial Intelligence Review Assistant (AIRA) was developed internally by Frontiers to address 4 targeted journal publication challenges:
- Improving the quality of manuscript technical and peer review. Internal teams perform tasks that are, as Mugridge emphasized, “only possible at scale through technological assistance” (described below).
- Reducing “reviewer fatigue.” Internal teams match manuscript topics to reviewers in Frontier’s database (based on Microsoft Academic) with appropriate expertise (described below).
- Matching editors to articles. Internal teams and chief editors use AIRA to identify appropriate handling editors.
- Connecting with funders. Frontiers provided funders with access to AIRA to find reviewers for COVID-19 funding proposals.
Hutt explained that AIRA “reads every manuscript” and produces a quality report “in just about a few seconds.” The report covers both manuscript and peer review quality items including plagiarism, language quality, controversial keywords, image manipulation, face detection, conflicts of interest (author and reviewer), and many others (Figure 1).
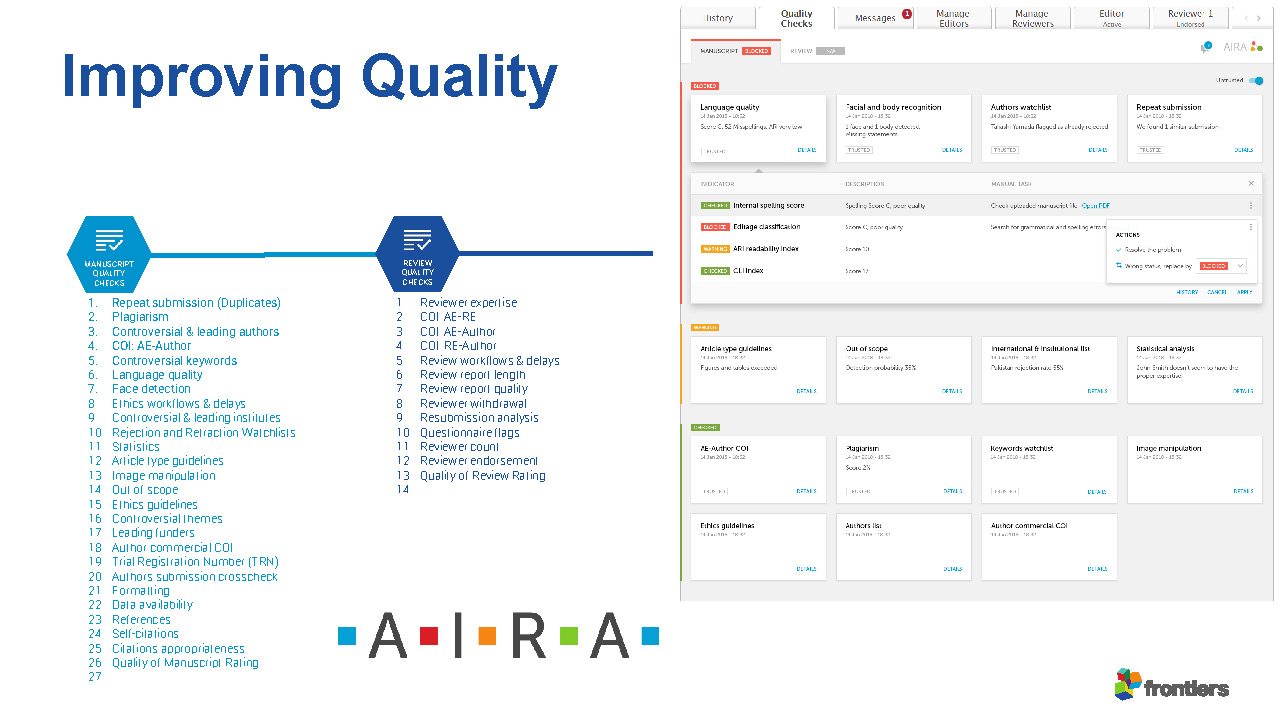
The report is then reviewed by the internal quality team; it is also available to editors, reviewers, and authors. (While the authors can see the full report before peer review, they are generally not encouraged to respond to the flagged items until after the manuscript has been reviewed.) Mugridge emphasized that rather than replacing reviewers or editors, AIRA “empowers them to make editorial decisions more effectively.”
For the reviewer match function, after the match is reported and evaluated, potential reviewers can be invited directly, along with an optional personalized email message. To assess some outcomes of the review-related functions, Frontiers compared internal data with Clarivate Analytics Global State of Peer Review survey data from 2018 and found a decrease (to 15%) in the rate of peer review invitations declined for out-of-expertise after the introduction of AIRA suggestions. The comparison also showed that review reports were 10% longer than the global average, and reviewers who were matched using AIRA submitted quality reports 3 days sooner than the average global reviewer.
Case 2: Piloting a Pre-Peer Review AI Technical Check Tool
UNSILO Evaluate Technical Checks is a machine learning- and natural language processing-based assisted editorial tool. ASCE is currently testing UNSILO Evaluate with 4 of their engineering journals. The UNSILO technical check is performed only on new submissions. The technical check is reviewed and approved by an internal quality team, and then the manuscript is sent to the chief editor. The items ASCE decided to focus on for their pilot study are the following:
- Word count
- Language/writing quality
- Figures and tables (all are included in the manuscript, and all are cited)
- Self-citation
- References (count, none are missing, and all are cited)
- Presence of the data availability statement
Chapman showed an example report and demonstrated how clicking on the flagged item in the report directs the evaluator to the place in the manuscript where the issue is located (Figure 2). Only the internal teams and chief editors see the report, which is part of the pre-peer review evaluation.
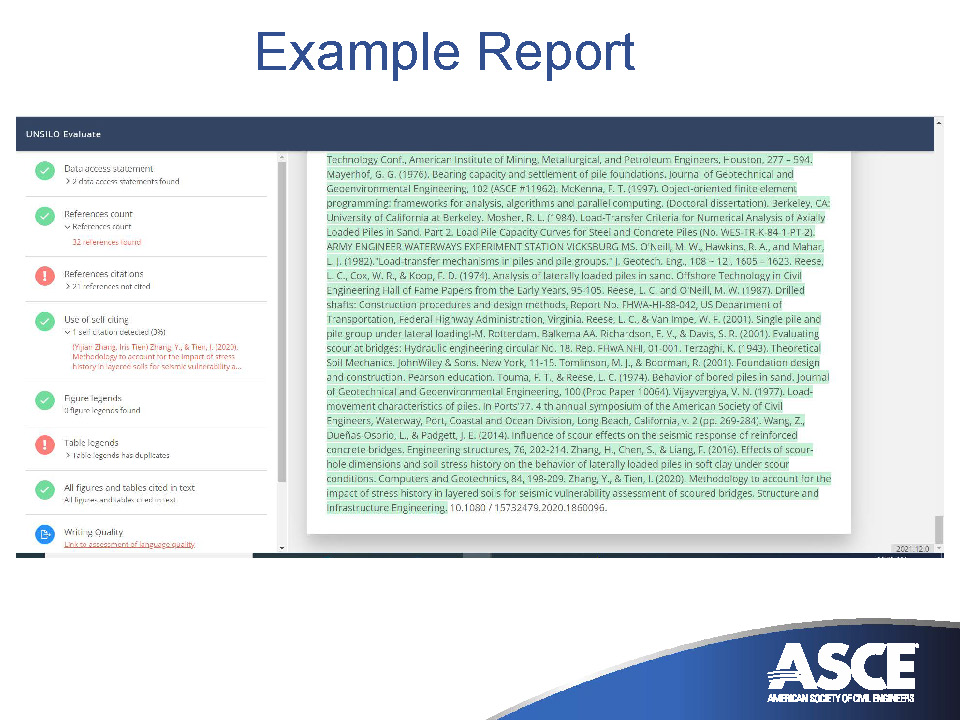
At this stage in the trial, the apparent benefits of the UNSILO tool are its utility for 1) providing guidance to editors for moving a manuscript through full review or referring the article for another disposition, 2) improving manuscript language, 3) citation checking, and 4) headlining/highlighting expedited editorial processes for article and journal promotion.
Chapman distilled the challenges ASCE has experienced with UNSILO Evaluate into the following general considerations for adopting AI-assisted editorial tools:
- Maintain good communication with the company that provides the tool. Working with them to identify and correct problems and improve the use of the tool benefits both the company and the client.
- Machine learning requires both positive and negative reinforcement to improve the tool’s reliability. You must continually provide adequate data. “Have enough data in your system to allow the [AI] system to learn.”
- Obtain editor feedback about the usability of the results and the helpfulness of the program. (What’s working best? What’s not working well? Is there something we should add to the program?)
- For efficient workflow, make sure the tool integrates well with the submission system in use.
- Budgeting for the AI system should include a cost/benefit analysis.
Case 3: Enabling Reproducible Reporting of Unique Resource Identifiers
One facet of scientific research reproducibility is the accurate and complete reporting of materials (such as antibodies, cell lines, organisms, software tools, and databases) with research resource identifiers (RRIDs). This was the target of the AACR SciScore pilot study.
SciScore is a machine learning-based public tool that analyzes the methods section of research articles. In the AACR SciScore pilot study, implemented across all 9 of the AACR journals, SciScore was integrated into the manuscript submission process. The authors receive the SciScore report within seconds of submitting their manuscript. They then have an opportunity to revise their methods section based on the results before their manuscript undergoes peer review (and they can use the results even if their report receives a desk rejection). Among other functions, SciScore identifies the reported resources and suggests appropriate RRIDs if the authors have provided sufficiently unique identifiers. The SciScore also includes, for clinical trials, a notification if the trial registration date is later than the study start date (Figure 3). Also, AACR includes instructions in their provisional acceptance letter asking the corresponding author to use the key resources table in the SciScore report to add RRIDs to their manuscript.
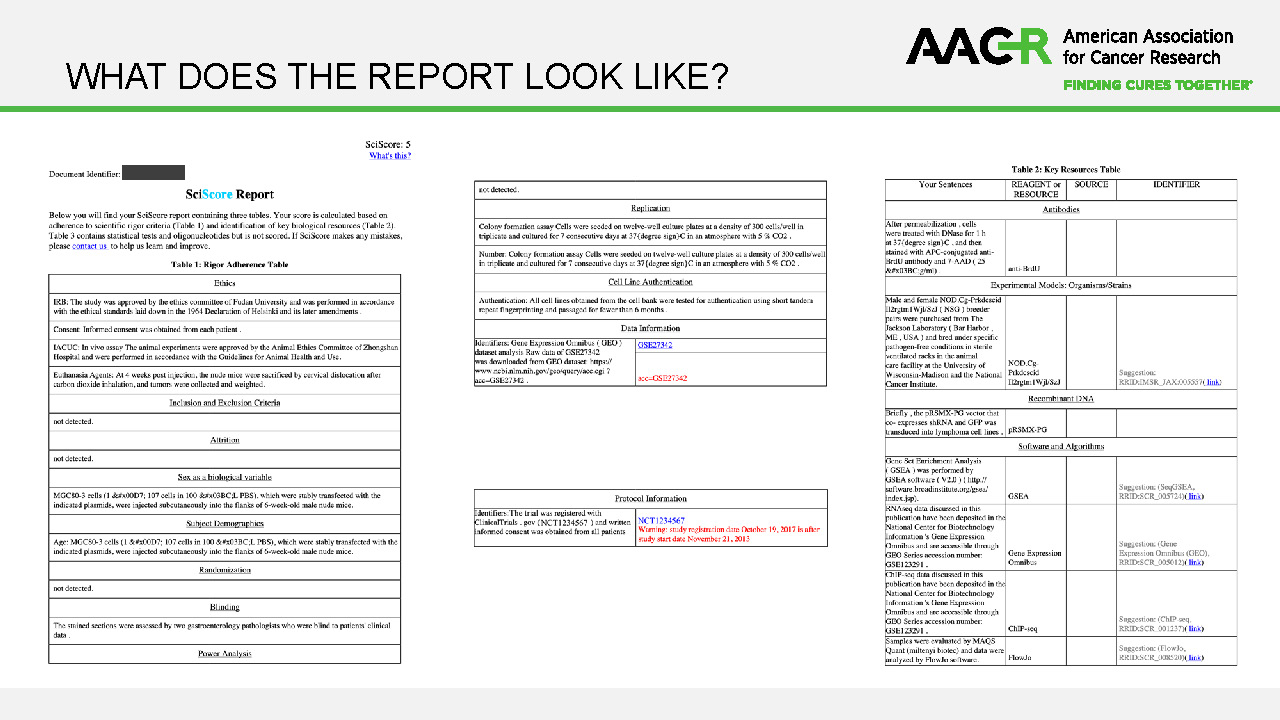
Preliminary results of the pilot study include that, with the implementation of the SciScore protocol, 10%–15% of authors revised the methods section of their manuscripts at least once during the submission, and those authors achieved a 1–2 point increase in their scores. The average daily SciScores increased quickly early in the study. Also, AACR observed consistent increases in the SciScore following peer review, which they attribute largely to the implementation of SciScore. To isolate the effect of the SciScore data from the effects of reviewer and editor comments, Evanko searched for correlated changes since AACR started the SciScore study. SciScore integration itself did not substantially affect the inclusion of RRIDs, but updating the provisional acceptance letter to prompt the author to add RRIDs from the SciScore report resulted in an immediate increase in RRIDs in the published articles.
Postpresentation Discussion
At issue in any discussion of AI-assisted editorial tools are concerns about confidentiality, costs, fairness/correcting inequities, and bias. For all of the speakers, transparency was a keyword: They all emphasized that 1) a useful AI-assisted editorial tool provides easily evaluable data; 2) the quality reports undergo periodic quality assessment themselves; and 3) all decisions are made by a person (not by a machine).
The effectiveness of the tools for assessing language quality was discussed during and after the case study presentations. At Frontiers, the most recent check for the language quality assessment function showed approximately 90% accuracy. The postpresentation discussion also touched on other potential sources of bias that may arise when using AI-assisted editorial tools, such as when applying filters for reviewer expertise. For example, AIRA’s software algorithms can be adjusted to select reviewers with a better expertise match, rather than filter for high h-indexes.
In response to Schultz’s question about how to respond to the concerns about the automation or checking of editors’ work, all speakers emphasized the limited, otherwise resource-intensive, technical nature of the tasks that AI-assisted editorial tools perform. Mugridge summarized this point by responding that the tools are designed to support editors, “not take over their jobs…Data-driven decision-making [by people]…is really key to any AI tool.”