Scholarly publishers—especially those in the scientific, technical, and medical fields—are increasingly enriching their content with an array of metadata with the aim of ensuring that content is distributed broadly, adaptable for multiple purposes, and rendered interoperable with other relevant content. Such metadata include digital object identifiers, ORCID identifiers, FundRef identifiers, PubMed links, GenBank sequence identifiers, and International Standard Name Identifiers. The options available continue to grow, and the value added to content grows as well. Semantic enrichment is an additional class of metadata that further improves the utility, discovery, and interoperability of content.
What Is Semantic Enrichment?
Semantic is often used in combination with terms such as enrichment, tagging, markup, indexing, fingerprinting, classification, and categorization. Although there can be important distinctions among these terms, they tend to be used loosely and interchangeably. In this article, we’ll use the catchall term semantic enrichment to refer broadly to the various technologies and practices used to add semantic metadata to content.
So, what is semantic enrichment?
A Topical Layer of Metadata
Added to Content Semantic enrichment is the process of adding a layer of topical metadata to content so that machines can make sense of it and build connections to it. Content in scientific articles and books is written so that humans can understand it, but computers have a hard time interpreting the nuances of human language. Given the explosion of available information— especially in the sciences—people have become reliant on computers to find the information that they need.
Semantic metadata provide the answer to an important question, “What is the meaning of this content?” in a way that computers can process so that they can find, filter, and connect information. Semantic metadata can be added to document markup (such as XML) to allow containers of information (such as journal articles, book chapters, guidelines, learning modules, and quizzes) to be broken into component parts so that the information can be acted on as distinct units of knowledge. Think of it as a content architecture through which a machine not only can understand that a chapter is made up of title, authors, sections, paragraphs, tables, figures, and so on but also can understand the topic and in some cases even the meaning conveyed by each component.
Once you have this topical map in the form of semantic metadata applied broadly across your content, you can automatically retrieve and organize information not just by its container but by its topic. For example, a medical publisher could pull together all relevant content on the topic of atrial fibrillation from all of its content types: journal articles, book chapters, clinical guidelines, continuing education, patient information, and more.
Semantically enriched XML is sometimes referred to as smart content because it holds within itself everything that an application needs to interpret it—both structurally and topically. Figure 1 shows the increasing value of content as increasingly rich markup is added.
How Is It Done?
The practice of adding semantic metadata to content is often called semantic tagging. A variety of technologies, methods, and practices can be used to enrich content with semantic metadata: Tagging can be embedded directly in XML files or can be held externally in databases or content management systems that reference elements in the content. For content that is not easily accessible, such as videos and images, tagging can be placed in metadata headers. More important than the exact method is that tags can be matched to specific elements in a document at the appropriate level of granularity.
Semantic tagging can be done at different levels of granularity in content. Tagging should be just granular enough to “atomize” content at a level that your customers will find appropriate and useful. Tagging can be done at the “top” of a container of content, for example, at the article level. Topic-collection tagging is one example of top-level semantic tagging. Tagging can also be applied deeper within a work; some systems tag major sections of a work, tables, and figures. Some go even deeper, tagging at the paragraph or even the sentence level. Named-entity recognition (also called entity extraction) is a granular form of semantic tagging that is used to identify predefined entities, such as persons, places, companies, clinical trials, drug names, gene sequences, and proteins. The right level of granularity for your organization and content will depend on how you intend to use the tagging.
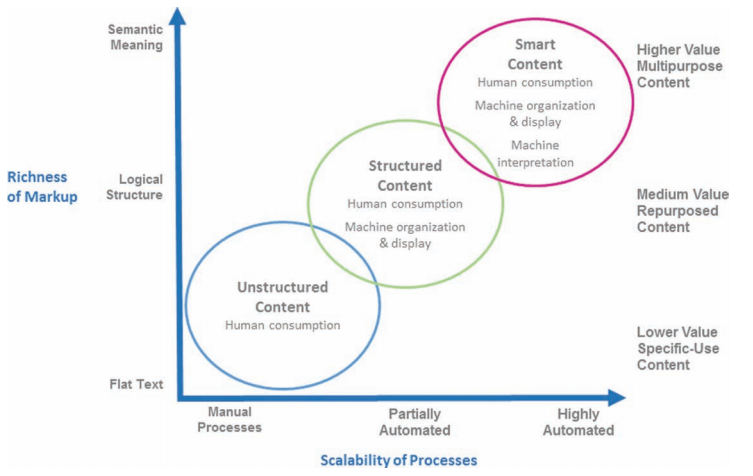
From “Smart content in the enterprise: How next-generation XML applications deliver new value to multiple stakeholders.” Published with permission. Copyright 2014 Outsell, Inc. www.outsellinc.com.
Who (or What) Tags?
Mechanisms for tagging content range from fully manual to fully automated.
In manual tagging, a person who has the appropriate expertise (sometimes called a subject-matter expert) reads the content and applies tags; this process is sometimes referred to as semantic indexing. Manual tagging is ideal when your intended use of tagging requires a high degree of precision, for example, in clinical applications such as clinical decision-support tools. But it can be cost prohibitive for large volumes of content because it is labor intensive and hard to scale to large volumes of work. Some content types, such as multimedia, are not amenable to automated systems, and manual tagging might be a better option.
In automated tagging, software analyzes content, adding tags on the basis of concept matching, statistical patterns, and linguistic analysis. Most automated systems include a “teaching” phase during which humans adjust the algorithms used for tagging to fit a specific data set and subject field and thereby increase the level of precision and accuracy that can be achieved through automation. Automated tagging is highly scalable and is good for finding trends in large bodies of content. It is sometimes the only option for very large content sets. However, automated approaches can lead to false positives (incorrect applications of a tag), missed concepts, and other inaccuracies.
Often, a hybrid approach is used—an automated process is followed by manual review and modification. For high-value, specialized uses (such as clinical decision-support tools that require “one best answer” results), this extra human touch may be necessary to achieve the right level of tagging accuracy.
Knowledge Organization Systems
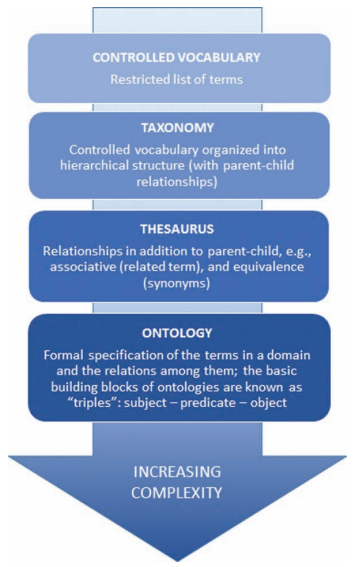
Figure 2 shows the different knowledge organization systems that can be used for content classification and organization. They range in complexity from a simple controlled list of common terms to a highly complex ontology that describes relationships between terms. Such classification systems are the framework for the semantic layer and semantic tagging. They control normalization, consistency in tagging, concept grouping and hierarchic relationships, and integrations and interoperability (both internal and external).
Industry Standard Knowledge Organization Systems
Your knowledge organization system must be able to interact with standards of your domain to forge useful external integrations. Many classification systems, usually in the form of taxonomies or thesauri, are in use in different scientific domains, such as the Unified Medical Language System (UMLS) and those from the American Chemical Society, American Institute of Physics, Association for Computing Machinery, Institute of Electrical and Electronics Engineers, Environmental Protection Agency, National Aeronautics and Space Administration, and US Geological Survey. Investigate what’s available in your scientific domain; if there is a system that is a good fit for your content and your intended uses, consider adopting it. A good example of a domainlevel knowledge organization system in medicine is the UMLS metathesaurus, which maps more than 100 healthcare vocabularies—for example, Medical Subject Headings (MeSH), Systematized Nomenclature of Medicine (SNOMED), and the International Classification of Diseases (ICD)—to support health-care interoperability.
If you are lucky enough to have an appropriate taxonomy or other classification system that describes your domain, make sure that you have a mechanism to adapt it to meet the needs of your content and your users and the pace of change and new concepts in your field. For science publishers in cutting-edge fields, a standard taxonomy will be unlikely to be updated fast enough to match your research output; you’ll need to be able to add concepts at the time of publication and reconcile them with the standard taxonomy later.
What Can Be Done with Semantically Enriched Content?
Once you have semantically enhanced your content, the benefits are many. A few are covered below.
Search and Discovery
Many publishers look to semantic enrichment to improve searching. Better search functionality makes users more productive, and this makes your content more useful to them. Time-strapped users are struggling with information overload, and fewer, better answers often are preferred. Your classification system should include equivalent relationships (also called nonpreferred terms), terms that essentially refer to the same thing. They can be synonyms, abbreviations, jargon, even misspellings. The equivalents can be used in your search to normalize the constantly evolving variations in the language that authors use to describe concepts and that searchers use to find them, allowing, for example, searches for “a-fib” to retrieve content on atrial fibrillation. Search “autocomplete” can also direct users to content by filling in matching concepts that are found in your content set as a user starts to type into the search box.
Semantic metadata also help to find non-text objects, such as images and videos, which can be tricky to find with full-text search because they contain little or no text to match on.
Topic Groupings and Hierarchic Relationships
In addition to serving as the “concept control” for tagging, semantic tagging governed by a taxonomy also allows content to be grouped topically—for example, to create topic collections or virtual journals—as well as hierarchically. A taxonomic hierarchy can even be provided to users in an application to allow them to broaden or narrow their exploration.
Related-Content Linking
Semantic tagging is a good way to offer users pathways for serendipitous discovery of related content—stumbling on gems that are highly relevant but that the user didn’t even know existed. Related-content linking allows a publisher to put additional relevant information in users’ paths and entice them to read more content; this can improve such metrics as number of page views and time on site. These links are dynamically generated as new content is added; new or updated links do not need to be “hard coded” as content is added.
Hooks for Integration and Interoperability
Semantic tagging can also provide “hooks” that allow you to connect external sources to your content and to exchange information across applications automatically. A good example of semantic tagging to provide integration hooks is the National Guideline Clearinghouse (NGC). NGC creates structured summaries of clinicalpractice guidelines and tags them with several health-care vocabularies, including ICD, MeSH, and SNOMED. This tagging enables external sources to connect to the guideline summary by using shared terms. For example, electronic health record (EHR) vendors can automatically provide guideline summaries within an EHR by using SNOMED terms.
It is increasingly important for publishers to integrate content into customers’ workflows to bring content to them in context as they do their daily work. Such customers might include clinicians at the point of care, researchers at the bench, or students preparing for an examination. Semantic tagging and domain standard classification systems can provide the hooks that allow your content to integrate with workflow applications.
Connecting Users and Content
Getting users to provide details of their interests when they register for site access is notoriously difficult. But as a user navigates content on semantically enabled sites, you can apply the tags on content visited to that user’s profile, eventually creating a profile that identifies the user’s interests. What topics is the user interested in? How are the user’s interests changing? Such user profiles can be used to create personalized information services or perhaps to connect users to communities of practice, groups of people who share an interest and who come together through social interaction to learn from each other.
Targeted Advertising
In addition to articles and book chapters that can be related through semantic tagging, advertising can also be related. Publishers can charge more for contextually targeted ads—ads that are topically related to content—than for nontargeted ads. Advertisers are increasingly interested in targeting ads to users instead of an article, and this is possible for sites that create user profiles through semantic tagging. Ads that are targeted to user profiles can be shown to users wherever they travel through the site.
New Products
Semantic enrichment lets you find topically related content and then recombine it to create new products from content that you already have. Such content recycling can lead to image collections, mashup and micro products that serve specialized audiences and fit specific workflows, and topically constructed objects, such as virtual journals, knowledge environments, coursepacks, and learning objects.
How to Get Started
What steps should you take to get started?
First, don’t start with technology. The temptation often is to jump into an exploration of the various technologies available and invite vendors in for demos. Before you explore technology, determine how you and your users will make use of semantic enrichment.
Create User Stories
Focus your semantic-tagging strategy on user stories. A user story captures what the user wants to achieve—who wants the functionality and why it allows that user to achieve something useful. How do people want to use your content? What tasks are they trying to do when they use your product? What answers are they looking for? At what point in their workflow is your content used? What content sets does it make sense to connect, both internally within your organization and with other content in your field or even related fields?
Your organization is also an important user of your product. What user stories does the marketing department have? Editorial? Advertising? For example, your advertising department might want to be able to target advertisements to related articles.
Even if your audience members are all part of the same specialty or are all members of your association, they will have different needs that depend on the roles that they are filling. A clinician wants to know the best treatment for the patient who is about to be seen. A researcher wants to know everything about a subject of interest. A student wants to prepare for an examination. Their user stories—and their demands of your content—will be different. If you solve a need for your users, you are more likely to create value and create successful features and products.
Measure Return on Investment
As with any investment in infrastructure, you need to consider the return on investment: Do the various benefits that accrue from semantic enrichment outweigh the costs? As is true of many enabling technologies, the return is not always straightforward. Just as in the case of an investment in an XML workflow, you will need to consider the various ways in which semantic enrichment will benefit your organization—increased content discovery and use through better search, browse, and related content linking; the ability to create new topically related products efficiently; increased user satisfaction; and premium rates from advertisers for ad targeting, to name just a few.
Be sure to look outside the publishing department for opportunities to connect content and users. Look to your organization’s overall digital strategy for clues. How can your semantic strategy support your organization’s overall goals? If you work in a society or professional association, for example, does your association have plans to integrate the professional content published in your books and journals with additional content available at your .org Web site, perhaps by connecting journal articles to relevant live events or other education programs? If so, using semantic enrichment to connect professional content with society programs will not only increase exposure and use of content but will also help your society to meet member needs.
Which Technology?
How do you decide which semantic technology to deploy? Focus on determining whether the technology supports your user stories. Here are some questions to ask when evaluating technologies and vendors:
- Does it offer or integrate with a constantly evolving knowledge organization system (such as a taxonomy)? How will it continually update tagging of your content to reflect new and changing terms?
- Does it meet the accuracy threshold for your users and your content?
- Can it tag at an optimal depth—both the right level of granularity and the right summary level?
- How will it handle figures, tables, video, and other media?
- Can the structure of the tagging output be supported by your existing content systems, in particular your Web content platform but also your content-management system, association management systems, and enterprise search?
Semantic Strategy
Semantic enrichment has many benefits, but issues of cost, scalability, and accuracy all complicate the technology decisions that need to be made, and all add risk. A well thought-out semantic strategy will maximize your probability of success. To develop your semantic strategy, focus on answering these questions:
- What are your organization’s user stories?
- What are the business benefits and the return on investment for your organization?
- What content do you need to tag, how is it delivered, and can the delivery systems and platforms use classification systems and tagging in a way that supports your user needs?
- What classification system will you use? Are standard taxonomies or thesauri available in your industry? What is your plan for keeping your classification system up to date?