Scientific publishing serves as a vital medium for sharing research results with the global scientific community. The images within an article are often integral to conveying those results clearly. However, with researchers sometimes including hundreds of sub-images in a manuscript, manually ensuring all images accurately depict the data they are intended to represent can be a challenge. Here, cancer researcher and founder of an artificial intelligence (AI) image-checking software tool,1 Dr Dror Kolodkin-Gal, explores how researchers and editors can improve image integrity, and how AI can streamline the publishing process.
The credibility and integrity of academic papers are of utmost importance. To maintain trust in scientific content, researchers, editors, and publishers have an ethical obligation to ensure all the data they share are valid. This is particularly significant when dealing with images or figures, which must be accurate to avoid misunderstanding, misinterpretation, and even allegations of deliberate image manipulation from readership. As more scientists use AI, image integrity will become increasingly important in scientific publication.
There are many forms of image integrity issues, but most of them are unintentional.2 An image may be mistakenly used twice, or researchers may use images containing overlaps. In any case, even a mistake in good faith can lead to an incorrect interpretation of the results and therefore must be avoided. At the same time, there are less common but much more severe cases in which there is deliberate manipulation. For example, deletion or addition of bands within western blots or cloning part of a microscopy image.
The Consequences
According to leading image data integrity analyst Jana Christopher, MA, the percentage of manuscripts flagged for image-related problems ranges from 20% to 35%.3 For authors, failing to detect image integrity issues before submission, either for grant requests or publication, can result in rejection. If a grant authority rejects a submission, it can delay access to funding, halting research. When reviewing complete papers, publishers do not need to disclose a reason for rejection during the peer review process; consequently, without receiving feedback, researchers may also be unaware that there are critical image issues in their manuscript.
If an issue goes undetected during review and is reported post-publication, either to the journal or online, the publisher and/or the author’s institution must investigate to determine if the allegation is true, how it occurred, and how to resolve it. Investigations can take years, during which time researchers may find it difficult to win further funding, conduct research, or publish elsewhere. While the image integrity issues may be unintentional, no matter the outcome of the investigation, researchers must work hard to rebuild their reputation.
In addition to costly investigations, image integrity issues can negatively impact future research. Academics often base new research on an existing paper—if the original paper contains inaccurate data, any data in new research will also be incorrect, wasting funding. Researchers may also find it difficult to replicate original results if they base their experimental procedures on an existing paper that contains errors, leading to more wasted time, materials, and funding.
Where Images Go Wrong
During an investigation, publishers will determine whether the flagged image issue is an intended manipulation or a mistake that went undetected. As mentioned earlier, when looking at the results of the investigations, we often find that many image issues are innocent mistakes.2 These mistakes are often instances of image duplication—this refers to reusing the same image in different parts of the paper without indication. The image may be used the same way twice, or may have been altered, for example, by changing the rotation, size, or scale. The image may have also been flipped or cropped, or researchers may use images containing overlaps.
Duplications can often happen when images are not effectively managed across time or when working in teams. Researchers may conduct experiments over many years, often collecting hundreds or thousands of images. Research can also be collaborative, with scientists from different universities working on the same project with a corresponding author. If these images are not properly managed, it might be difficult to distinguish between them and errors can occur.
So, why do these issues occasionally go undetected? Publishers and editors have large numbers of manuscripts awaiting attention after submission, whether pre- or post-peer review. The sheer volume of manuscripts submitted, combined with pressures such as time constraints makes ensuring image integrity difficult, particularly because editors must manually check each image and compare them with the rest of the paper—this presents a significant challenge.
AI in Research
Maintaining image integrity and the reliability of the scientific literature in general has become more complicated since the recent proliferation of the use of AI.
Globally, the advance of AI has led to developments in a wealth of varied applications, from autonomous vehicles to virtual assistants. In scientific research and publishing, it is no different. Developments in AI technology can help researchers, editors, and publishers in life sciences improve not only the ease of writing and editing a manuscript, but also can increase the integrity of their work and the impact of their publications.
For instance, AI-based grammar and plagiarism checking tools have advanced far beyond a simple spell-check. They now enable researchers to review written content for clarity, originality, and the proper citation of sources. These tools can analyze text for tone and provide suggestions for improvements, ensuring that the language used aligns with the intended context and scientific rigor.
Content Generation
While there are multiple benefits to using advanced tools, the integration of AI in scientific publishing brings forth ethical considerations that demand thoughtful evaluation. While few people would suggest that using AI for spell-checking was inappropriate, the use of AI for content generation is now as controversial as it is popular.
Although AI-generated content can provide valuable insights, such as by summarizing lengthy documents, it is essential to acknowledge that these systems are only as good as the data they are trained on. Biases or inaccuracies present in the training data can manifest in the generated content, potentially leading to misinformation.
For example, the first AI generated article in Men’s Health magazine was investigated for sharing many inaccuracies and falsehoods—despite the fact the content appeared to have academic-looking citations.4
To maintain integrity and trust in scientific research, it is crucial to apply transparency, accountability, and credibility to the use of AI in content generation. Some publishers have already adapted their editorial policies to restrict the use of large language models like ChatGPT in scientific manuscripts to prevent misinformation.
The limitations of AI’s performance and transparency mean that human intervention remains indispensable. Perhaps more than ever, researchers and editors should continue to verify facts and exercise due diligence during peer review to ensure that AI-generated content aligns with scientific rigor.
Paper Mills
Mistakenly sharing inaccurate content is one risk, but AI’s integration into scientific publishing poses another ethical challenge: the expansion of paper mills. These organizations, that produce entirely fabricated content, highlight how AI can be exploited to undermine the credibility of scientific publications.
The exact percentage of paper mill articles in circulation is unknown. The Committee on Publication Ethics conducted a study that suggested the percentage of suspect papers being submitted to journals ranges between 2% and 46%.5 Significant concerns exist among publishers that this difficult-to-detect phenomenon undermines the credibility of scientific publications.
Advancements in generative AI may enable paper mills to produce seemingly more sophisticated and authentic content. Generative text and image algorithms can read existing scientific literature to mimic research writing styles, rewrite existing content, or generate pseudo-scientific articles and images that resemble genuine material. The figure, for example, demonstrates content generated by AI image generation tool DALL·E when giving prompts.
The more closely AI can mimic authentic content, the more difficult it will be for publications to detect intentionally fraudulent submissions. The fact that generative AI can produce material so rapidly could increase the scale of the problem, placing a higher burden on journal editors.
Forensic editors play a crucial role in ensuring the integrity and credibility of scientific research by detecting and addressing any allegations of misconduct and fraud. Forensic editors will review the paper’s contents and data, gather evidence, and interview relevant parties to assess the validity of any reports of fraud. This will become increasingly difficult as AI technologies become more sophisticated.
Find AI with AI
However, work is underway to tackle this growing challenge. While some AI tools are prone to misuse by paper mills, others could be part of the solution to detecting and preventing the publication of fraudulent research. Some researchers are already using machine learning to develop algorithms that could check for a range of signals that suggest paper mill content.6
Several AI image detectors are also now available, such as Maybe’s AI Art Detector,7 or AI or Not.8 However, these tools are not 100% accurate; one concluded that the images in the Figure were generated by a human, and one recognized them as AI-generated.
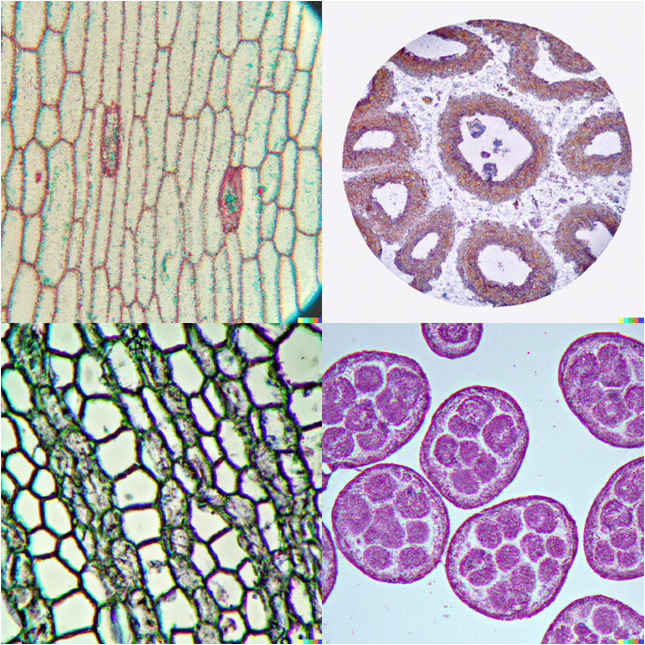
Over the years, a range of characteristics have been found that may distinguish paper mill articles. These encompass not only the text, but also the scientific images used in a paper. However, manually reviewing papers for suspected image manipulation can be a time-consuming task that is not always accurate, particularly because a paper could include hundreds of sub-images.
For example, by using cut and paste or certain filters on images, authors willing to manipulate research, or a paper mill organization, can alter results and make a manuscript appear more authentic. These forms of image issues are often difficult to detect by eye and compare against existing imagery. Comparing potentially manipulated images against a database of millions of previously published pictures might prove futile because AI-created images could appear authentic and unique, despite the lack of legitimate data.
It is clear that integrity experts can no longer rely purely on manual checks and must consider countermeasures to AI misuse. Identifying these sophisticated fakes is a significant challenge. AI Computer Vision—the field of AI that trains computers to analyze and interpret the visual world using digital images—can automate the review process to detect image issues before publication. Image integrity proofing software tools use computer vision and AI to scan a manuscript and compare sub-images in minutes, flagging any potential issues. Editors can then investigate further, using the tool to find instances of cut and paste, deletions, or other forms of manipulation as well as instances of innocent duplications. The editor can then decide how to proceed with the paper.
AI has many capabilities and will continue to improve, but we cannot rely on technology to act ethically of its own accord. As the scientific community increases its understanding of AI and its applications, integrity experts should collaborate to establish clear guidelines and standards for its use in content generation.
Yet, despite these efforts, paper mills and fraudulent image manipulation will persist. However, it is important to note that while deliberate manipulation of images poses a significant challenge to image integrity in scientific publishing, the vast majority of image-related issues still stem from honest mistakes. Publishers, therefore, should continue to invest in and adopt the most suitable technological solutions available at the time for reviewing manuscripts prior to publication. This, of course, should be complemented by a widespread endeavor to develop additional methods to prevent the flourishing of paper mills and manipulated manuscripts.
As we navigate this transformative era, collaboration and responsible AI usage will pave the way for a future where scientific publishing remains a beacon of trust and integrity.
References and Links
- https://www.proofig.com/?utm_source=Stone+Junction&utm_medium=Commission+&utm_campaign=Science+Editor+&utm_id=PRO098&utm_content=Earned+
- Evanko DS. Use of an artificial intelligence–based tool for detecting image duplication prior to manuscript acceptance. Ninth International Congress on Peer Review and Scientific Publication, September 8–10, 2022, Chicago, IL. [accessed January 21, 2024]. https://peerreviewcongress.org/abstract/use-of-an-artificial-intelligence-based-tool-for-detecting-image-duplication-prior-to-manuscript-acceptance/
- UKRIO. Expert interview with Jana Christopher, MA, Image Data Integrity Analyst. [accessed January 21, 2024]. https://ukrio.org/ukrio-resources/expert-interviews/jana-christopher-image-integrity-analyst/
- Christian J. (2023) Magazine publishes serious errors in first AI-generated health article, Futurism. Neoscope, 2023. [accessed January 21, 2024]. https://futurism.com/neoscope/magazine-mens-journal-errors-ai-health-article
- Committee on Publication Ethics, STM. Paper mills: research report from COPE & STM. 2022. [accessed January 21, 2024]. https://doi.org/10.24318/jtbG8IHL
- STM. STM Solutions releases MVP of new paper mill detection tool, STM. 2023. [accessed January 21, 2024]. https://www.stm-assoc.org/papermillchecker/
- https://huggingface.co/spaces/umm-maybe/AI-image-detector
- https://www.aiornot.com/
Dr Dror Kolodkin-Gal, co-founder, Proofig (https://www.proofig.com/).
Direct press enquiries to Alison Gardner (alison@stonejunction.co.uk) and Leah Elston-Thompson (leah@stonejunction.co.uk) at Stone Junction.
Opinions expressed are those of the authors and do not necessarily reflect the opinions or policies of the Council of Science Editors or the Editorial Board of Science Editor.